Research
Data-driven decision support in logistics and healthcare
The main research areas of the chair are briefly described below. Operations research and data analytics methods are used individually or in combination in all areas. In home delivery, to improve the efficiency and service of last mile delivery, and in healthcare, to reduce treatment costs and improve patient health. Please note that this page is only an overview of existing and therefore expandable research questions for the future.
For many goods ordered online, it is necessary for the customer to be present in person at the time of delivery. This is particularly the case with online supermarkets and fresh food. For example, the milk ordered should be placed in the fridge quickly and must not be left with a neighbour or elsewhere.
To make this possible, online supermarkets offer their customers a choice of delivery windows. However, it is a challenge for retailers to organise these time slots in such a way that they are both attractive to customers and cost-efficient. Customers generally prefer short delivery windows, often less than an hour. However, short time windows lead to more complex route planning, which increases delivery costs. In addition, the retailer does not know when customers want to be delivered. If a customer places an order and the retailer makes a delivery promise for a time window, this may no longer be a good decision a short time later if another customer places an order and the delivery route changes.
The aim of the retailer is to serve as many customers as possible in order to operate economically. However, there is a limited capacity of customers that can be accepted, for example due to the available number of drivers and delivery vehicles.
In our research, we are investigating how retailers can be supported in making decisions about acceptance and time slot offers. Here we try to estimate the expected delivery costs more accurately or use historical data to predict the expected order quantity. This should help to make deliveries more efficient and customer-friendly.
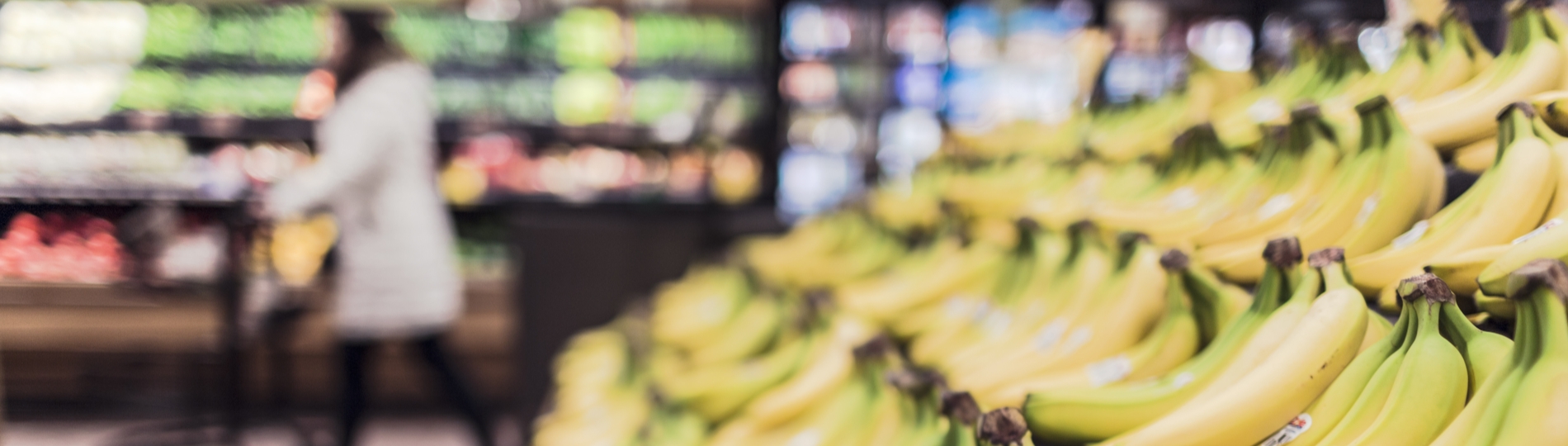
In the field of mental health, many innovative technologies promise to improve care, including apps to support patients. Although they offer a potential increase in the quality of care, they are often criticised because their benefits are considered too low and their costs too high. However, an economic evaluation of such innovative technologies - based on health outcomes and costs - can more clearly demonstrate the actual benefits and thus promote their faster acceptance.
Our research focuses on analysing health and cost aspects of traditional treatments compared to IT-based approaches for patients with mental illness, for example using simulation. In this way, we explore potential financial savings or benefits for hospitals and mental health service providers, as well as the improvement of mental health care for patients.
Smartwatches have evolved into powerful trackers that can record a wide range of health data, including heart rate, sleep quality and oxygen levels. Some even offer the option of recording an ECG directly on the wrist. These features open up the possibility of enhancing patient care through the integration of smartwatch data, which can lead to a more detailed understanding of health status.
From patients bringing printed copies of their own vital signs to their doctor's appointment (a feature that many smartwatches already support) to the integration of this data into medical systems (such as patient records), there are numerous potential applications. This new availability of data offers the potential to improve healthcare, for example through the use of artificial intelligence to predict future diseases.
Our research focuses on how this data integration can improve the treatment process and how it can be used to predict and prevent diseases. Our main goal is to maximise the potential of wearables to improve healthcare.
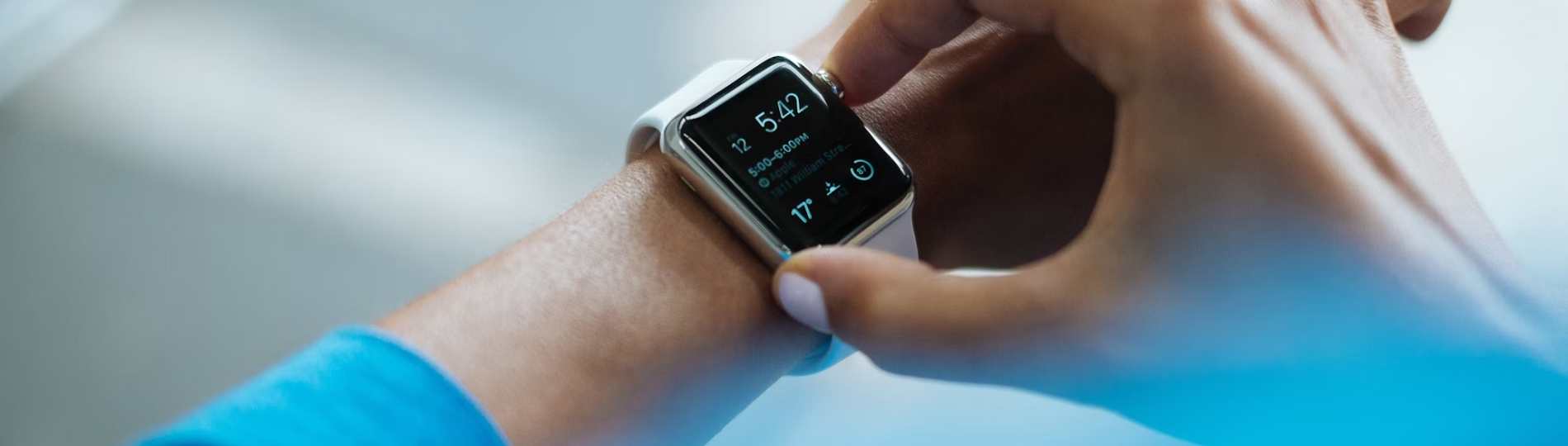